Revolutionize Resource Allocation with AI Project Agents: Maximizing Efficiency
In today’s fast paced business world, resource allocation is key to project success. AI project agents are the game changers in this space, using advanced algorithms, predictive analytics and automation to optimize efficiency, reduce waste and dramatically improve project outcomes.
Introduction to AI-Driven Resource Allocation
AI-driven resource allocation is revolutionizing the way organizations manage their resources. By leveraging advanced algorithms and machine learning techniques, AI systems analyze vast amounts of data to make informed decisions that enhance efficiency, reduce waste, and improve overall performance. This innovative approach allows organizations to optimize the distribution of resources across various sectors, leading to increased productivity, cost savings, and better decision-making. Integrating AI into resource management processes ensures that resources are allocated where they are needed most, maximizing their value and impact.
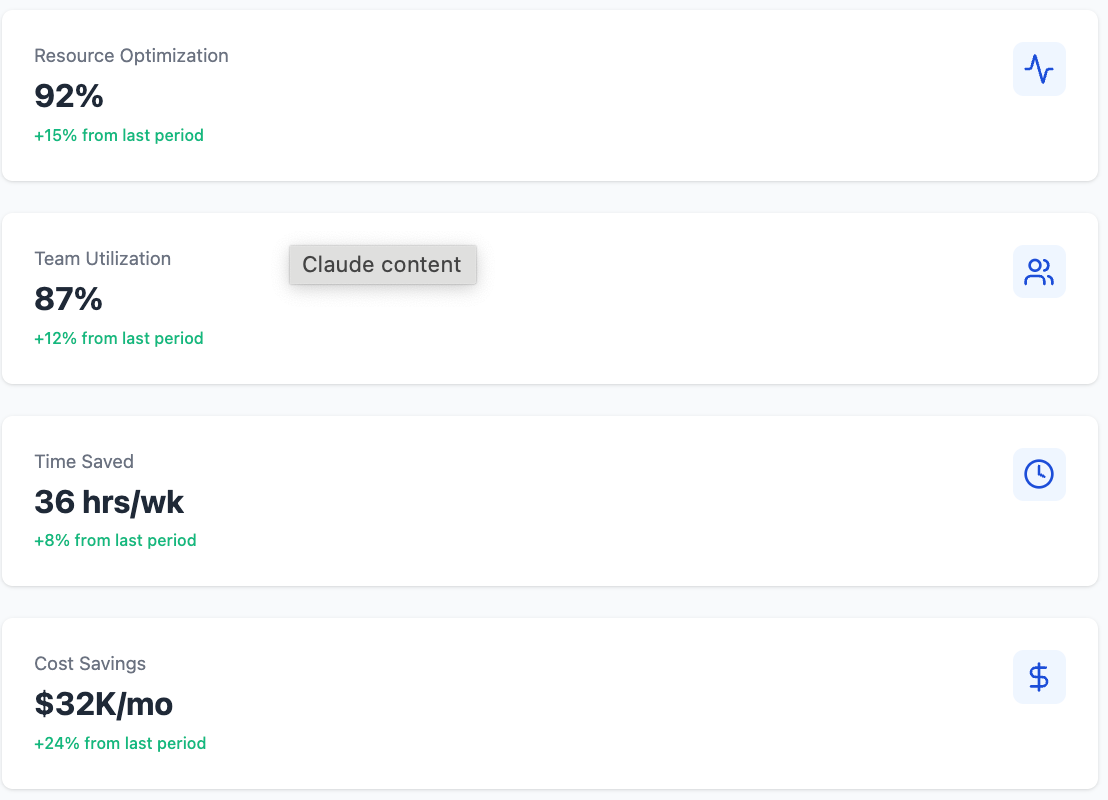
Defining Resource Allocation
Resource allocation is the strategic process of distributing available resources—such as financial assets, human capital, technology, and time—among various projects, departments, or tasks within an organization. Effective resource allocation ensures that the right resources are assigned to the right tasks at the right time, optimizing performance and helping organizations achieve their strategic goals. By carefully managing resources, organizations can operate more efficiently and effectively, ultimately driving better outcomes and achieving their objectives.
The Resource Allocation Problem
Traditional resource allocation methods rely on manual processes and historical data, resulting in inefficiencies, bottlenecks and suboptimal utilization. AI project agents solve this problem by bringing intelligence and automation to the entire resource management lifecycle.
The Role of AI Agents in Resource Allocation
AI agents play a pivotal role in optimizing resource allocation by automating routine tasks and allowing team members to focus on more strategic activities. These intelligent systems excel at monitoring resource availability and capacity in real-time, continuously analyzing data from various sources to allocate resources efficiently. By evaluating current data and adjusting project priorities, AI agents ensure that critical tasks receive the necessary resources, thereby enhancing overall project performance and resource utilization.
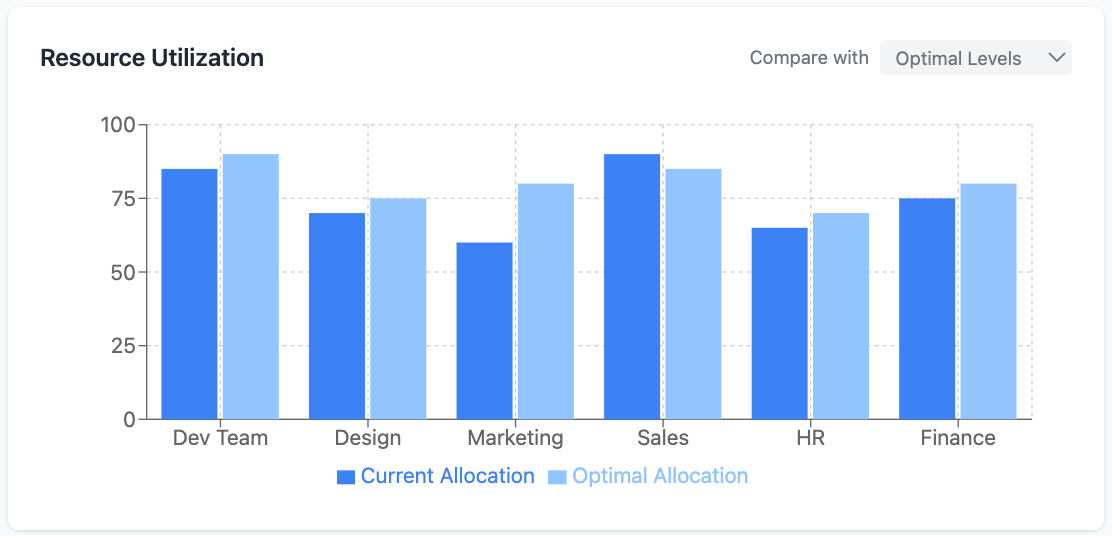
AI vs. Traditional Methods
AI-driven resource allocation offers significant advantages over traditional methods. Unlike manual processes, AI systems can analyze vast amounts of data, identify patterns, and provide insights that human managers might overlook. AI agents can predict future resource demands, helping project managers plan and allocate resources more effectively. Additionally, AI agents make decisions based on real-time analysis, continuously learning from data and dynamically adapting their strategies. This ability to process and act on real-time data ensures that resources are used optimally, reducing inefficiencies and improving project outcomes.
Key Benefits of AI Project Agents in AI Driven Resource Allocation
Optimized Efficiency
AI ensures resources are allocated where they deliver the most value. Waydev shows how AI algorithms minimize idle time and maximize productivity by matching resources to tasks based on multiple variables at once. This level of optimization is impossible with manual methods.
Better Decision Making
Project managers have to make countless resource allocation decisions under uncertainty. IMT Project Management explains how AI’s predictive analytics can simulate different allocation scenarios, so managers can make decisions based on real time data and projected outcomes.
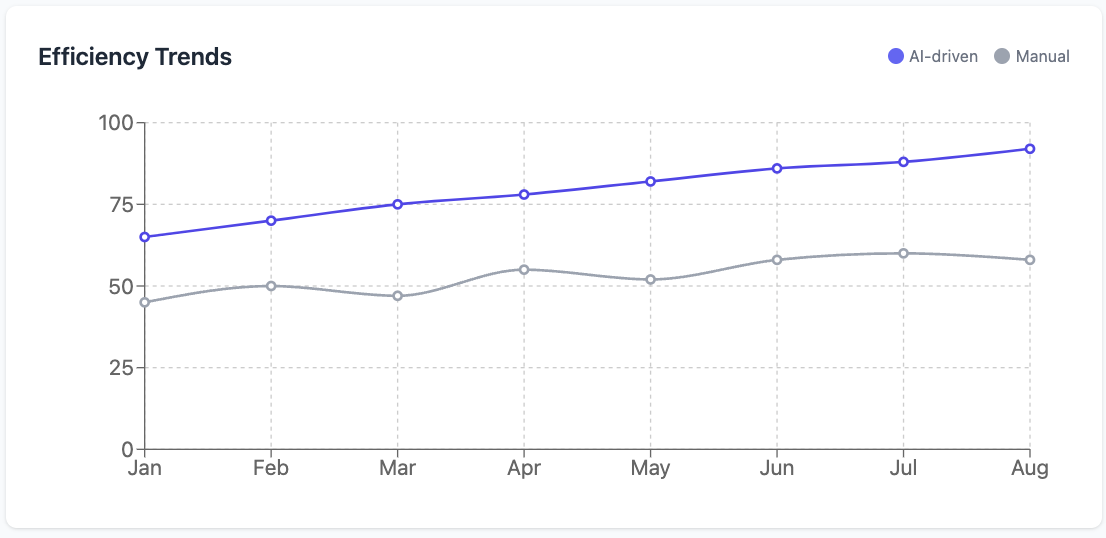
Cost Savings
In resource intensive projects, inefficiencies translate to budget overruns quickly. ClickUp shows how AI agents reduce waste and optimize budgets by finding cost saving opportunities while maintaining project quality and delivery timelines.
Flexibility
Projects never go exactly as planned. AI project agents adjust resource allocation processes based on changing priorities or unexpected challenges. Rapid Innovation demonstrates how this adaptive approach keeps projects on track despite changing conditions.
Automation of Routine Tasks
Resource allocation involves many repetitive tasks like scheduling, assignments and data analysis. LeeWay Hertz shows how AI automates these mundane activities so project teams can focus on strategic decisions and creative problem solving.
Real Time Insights
Traditional resource allocation suffers from information delays. AI agents provide continuous monitoring of resource availability and utilization so you can make proactive adjustments to prevent bottlenecks and delays before they impact project timelines.
Technical Architecture of AI Resource Allocation Agents
The technical architecture of AI resource allocation agents is composed of three main layers: the Data Ingestion Layer, the Machine Learning Layer, and the Decision-Making Layer. The Data Ingestion Layer collects and processes relevant data from various sources, ensuring that the AI system has access to accurate and up-to-date information. The Machine Learning Layer analyzes this data, using advanced algorithms to make predictions about future resource needs. Finally, the Decision-Making Layer uses these predictions to make informed decisions about resource allocation, ensuring that resources are distributed efficiently and effectively.
Machine Learning Fundamentals
Machine learning (ML) is a subset of artificial intelligence (AI) that focuses on developing algorithms that enable computers to learn from and make predictions based on data. There are three main types of machine learning algorithms: Supervised Learning, Unsupervised Learning, and Reinforcement Learning. Supervised Learning involves training algorithms on labeled data, allowing them to make accurate predictions. Unsupervised Learning involves discovering patterns in unlabeled data, helping to identify trends and insights. Reinforcement Learning involves training algorithms through trial and error, enabling them to learn from their actions and improve over time. These machine learning techniques are fundamental to AI-driven resource allocation, allowing systems to analyze data, predict resource needs, and optimize resource usage.
Use Cases Across Industries
Dynamic Scheduling and Task Assignments
AI is great at matching team members to tasks based on their skills, availability and current workload. This ensures optimal utilization of human resources and prevents overallocation and underutilization of team members.
Predictive Analytics for Capacity Planning
One of the biggest challenges of resource management is forecasting future needs. AI analyzes historical data and current resource usage patterns to predict resource requirements for upcoming projects so you can plan hiring, training or procurement well in advance.
Budget Allocation
Aisera shows how AI agents allocate budgets across departments or projects with incredible efficiency, analyzing spending patterns to find cost saving opportunities while keeping critical initiatives funded.
Supply Chain Management
In manufacturing and construction projects, AI predicts material needs and coordinates with suppliers to ensure timely delivery without overstocking or shortages. This just-in-time approach minimizes inventory costs and prevents project delays.
Real Time Resource Re-allocation
When priorities change or unexpected challenges arise, AI can re-allocate resources during project execution. SAP shows how this ensures the most critical tasks always have enough resources without manual intervention.
Scenario Planning
Before committing to a resource allocation strategy, AI can simulate the impact of different approaches. This allows project leaders to choose the best strategy to achieve project goals under different constraints and conditions.
IT Resource Management
In technology environments, AI allocates server, network and cloud resources based on demand patterns. Google Cloud shows how this prevents downtime while optimizing performance and controlling cloud spend.
Implementation Tips
Organizations looking to use AI for resource allocation should consider these tips:
Start with clear objectives - Define what optimal resource allocation means for your specific use case
Ensure data quality - AI agents rely on historical and real time data
Integrate with existing tools - Connect AI agents with your project management and resource planning systems
Start with hybrid approaches - Combine AI recommendations with human oversight as you build confidence
Measure impact - Track improvements in resource utilization, project timelines and budget adherence
The Future of AI in Resource Allocation
As AI technology advances, we can expect even more advanced capabilities in resource allocation. Emerging trends include:
- Cross project optimization - AI that manages resources across an entire portfolio of projects
- Self learning allocation models - Systems that improve automatically based on outcomes
- Advanced constraint handling - AI that balances complex and sometimes contradictory allocation requirements
- Natural language interfaces - Ability to adjust resource allocation through conversational commands
Conclusion
AI project agents are fundamentally changing resource allocation by combining automation with intelligent decision making. Organizations that adopt these technologies can streamline resource management, improve team collaboration and achieve better project outcomes with fewer resources.So, get started now.